Electrical Resistance Tomographic by Using Current Injection and Magnetic Field Induction
DOI:
https://doi.org/10.25299/jgeet.2023.8.1.10560Keywords:
III-posed, sensitivity, current injection, magnetic field induction, reconstruction imageAbstract
A critical issue in electrical tomography is ill-posed problems due to low sensitivity. In the electric current injection method, the placement of the injection electrode on the object boundary can influence it. This condition causes the reconstruction result of parameter change far away from the boundary to be inferior in quality. Another excitation method is using magnetic field induction proposed to overcome these problems. Each reconstruction image was obtained using two methods with three types of parameter changes, that represented the edge and the center of the object position. Both reconstruction results are merged and further processed to enhance the quality of the image, based on the average value of the resistivity of each element. The results show that the final image reconstruction has a smaller root mean square error (RMSE) than the electric current injection method.
Downloads
References
Alsaker, M., Hamilton, S. J. and Hauptmann, A. (2017) ‘A direct D-bar method for partial boundary data electrical impedance tomography with a priori information’, Inverse Problems and Imaging, 11(3), pp. 427–454.
Arai, K. (2020) ‘Image restoration based on maximum entropy method with parameter estimation by means of annealing method’, International Journal of Advanced Computer Science and Applications, 11(8), pp. 255–261.
Chitturi, V. and Farrukh, N. (2017) ‘Spatial resolution in electrical impedance tomography: A topical review’, Journal of Electrical Bioimpedance, 8(1), pp. 66–78.
Darbas, M. et al. (2021) ‘Sensitivity analysis of the complete electrode model for electrical impedance tomography’, AIMS Mathematics, 6(7), pp. 7333–7366.
Darmawan, D. et al. (2015) ‘Study of Induced Current Electrical Impedance Tomography Configuration on 2 Dimensional Rectangular Object’, Proceedings of the 2014 International Conference on Physics and its Applications, 1(June 2016).
Darmawan, D. et al. (2016) ‘Applied current injection and magnetic field induction simultaneously on electrical impedance tomography’, International Journal of Tomography and Simulation, 29(1).
Deddy Kurniadi, J. (2014) ‘Electrical Impedance Tomography in Rectangular Object Using Data Collection System Based on Absolute Boundary Potential Measurement’, Mycobacterial Diseases, 04(02), pp. 2–6.
Dingley, G. and Soleimani, M. (2021) ‘Multi-frequency magnetic induction tomography system and algorithm for imaging metallic objects’, Sensors, 21(11).
Feldkamp, J. R. and Quirk, S. (2019) ‘Internal magnetic induction tomography using a single coil’, Progress in Electromagnetics Research, 164(December 2018), pp. 97–107.
Gong, B. et al. (2016) ‘EIT Image Reconstruction by Modified Data’, Journal of Biomedical Science and Engineering, 09(10), pp. 99–106.
Harikumar, R., Prabu, R. and Raghavan, S. (2013) ‘Electrical Impedance Tomography (EIT) and Its Medical Applications : A Review’, International Journal of Soft Computing and Engineering (IJSCE), 3(4), pp. 193–198.
Khan, T. A. and Ling, S. H. (2019) ‘Review on electrical impedance tomography: Artificial intelligence methods and its applications’, Algorithms, 12(5).
Kumar, K. et al. (2016) ‘Content Based Image Retrieval Using Gray Scale Weighted Average Method’, International Journal of Advanced Computer Science and Applications, 7(1), pp. 1–6.
López C., D. C. et al. (2015) ‘Nonlinear ill-posed problem analysis in model-based parameter estimation and experimental design’, Computers and Chemical Engineering, 77, pp. 24–42.
Ma, L. and Soleimani, M. (2017) ‘Magnetic induction tomography methods and applications: A review’, Measurement Science and Technology, 28(7).
Ma, L., Wei, H. Y. and Soleimani, M. (2013) ‘Planar magnetic induction tomography for 3D near subsurface imaging’, Progress in Electromagnetics Research, 138(March), pp. 65–82.
Noushad, M. (2017) ‘Image Pair Fusion using Weighted Average Method’, 3(10), pp. 397–402.
Rane, N. D., Kakde, P. B. and Jain, P. M. (2017) ‘Comparative study of Image Fusion Methods : A Review’, International Journal of Engineering and Applied Sciences, 4(10), pp. 67–72.
Seppänen, A. et al. (2009) ‘Electrical resistance tomography imaging of concrete’, Concrete Repair, Rehabilitation and Retrofitting II - Proceedings of the 2nd International Conference on Concrete Repair, Rehabilitation and Retrofitting, ICCRRR, pp. 231–232.
Wang, J. et al. (2018) ‘Magnetic Induction Tomography Simulation Analysis Based on Comsol Multiphysics Soft’, IOP Conference Series: Materials Science and Engineering, 394(4).
Wang, Y. (2020) ‘Image segmentation method with maximum entropy optimized by Wolf pack algorithm’, Journal of Physics: Conference Series, 1650(3).
Zhao, Z., Li, X. and Cheng, H. D. (1993) ‘Image enhancement using fuzzy logic’, International Conference on Fuzzy Theory and Technology Proceedings, Abstracts and Summaries, pp. 74–75.
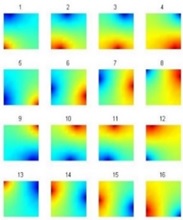
Downloads
Published
Issue
Section
License
Copyright @2019. This is an open-access article distributed under the terms of the Creative Commons Attribution-ShareAlike 4.0 International License which permits unrestricted use, distribution, and reproduction in any medium. Copyrights of all materials published in JGEET are freely available without charge to users or / institution. Users are allowed to read, download, copy, distribute, search, or link to full-text articles in this journal without asking by giving appropriate credit, provide a link to the license, and indicate if changes were made. All of the remix, transform, or build upon the material must distribute the contributions under the same license as the original.